Learning Lipids Part V: The Lipid Lifestyle
Uncovering how different lifestyle interventions impact lipid metrics and CVD risk.
Last time in the Learning Lipids Series, we began a discussion around what I referred to as the, “heroes,” of the lipidology story, as we explored some of the pharmacological interventions for reducing Apo-B particle count and managing CVD risk. This week we will explore the second flavor of approaches to cleaning up lipid metrics and cardiovascular health: lifestyle interventions. This body of literature is vast, diverse, and dense, so this is not an exhaustive review of the lifestyle mediums and modalities that impact CVD risk – for example, I do not mention anything regarding exercise and CVD risk; rather, it is a display of some of the research I found to be interesting, actionable, and impactful from this field. Now, if you’re just joining this lipid party or need a refresher, check out the previous parts of the series (I, II, III, IV) and/or the recap below. Now, here is Learning Lipids Part V, a deep dive on how lifestyle interventions impact lipid metrics and CVD risk.
Medical Disclaimer: I am not a doctor, and none of the information below is medical advice in any way, shape, or form. Let this post serve as a spark to your curiosity and a stepping stone to conversations with your personal medical providers.
Preface: In order to fit this post into email format, I only included hyperlink citations, rather than including the full reference list; however, you can find that complete reference list here.
Recap
Cholesterol is a type of lipid, a compound that is nonpolar and does not dissolve in water as a consequence. In order to transport cholesterol and other lipids, your body packages them into protein complexes, called lipoproteins, composed of polar exteriors.
Lipidology can be simplified to the analogy of a highway, where the route represents a blood vessel, the vehicles represent lipoproteins, and the contents carried within/on the vehicles represent lipids.
The LDL lineage is broken down based on density, and each particle’s protein-to-lipid ratio determines its density, in that the greater amount of lipids within the particle, the less dense the particle is and vice versa.
Very low-density lipoproteins (VLDLs) sit at the least dense end of the spectrum, as they contain the most amount of triglycerides and cholesterol. As those VLDLs offload lipids to cells throughout the body, they become denser and denser, until they are dense enough to be categorized as intermediate-density lipoproteins (IDLs). The process continues, as the IDLs ultimately relinquish their lipids, increase in density, and become low-density lipoproteins (LDLs).
Since the late 1950s, scientists and physicians have looked to cholesterol-based metrics to assess CVD risk; however, in recent years, cardiologists and lipidologists, like Allan Sniderman and Tom Dayspring, have challenged the status quo of CVD risk assessment, pointing to lipoprotein particles count as the best predictor of risk.
Since the size and cholesterol concentration of LDL particles varies, multiple LDL particle concentrations can produce the same LDL cholesterol concentration (as shown in Images B and C below).
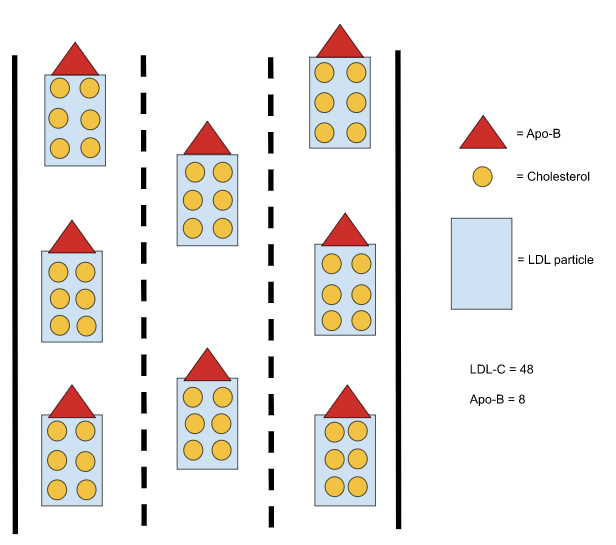
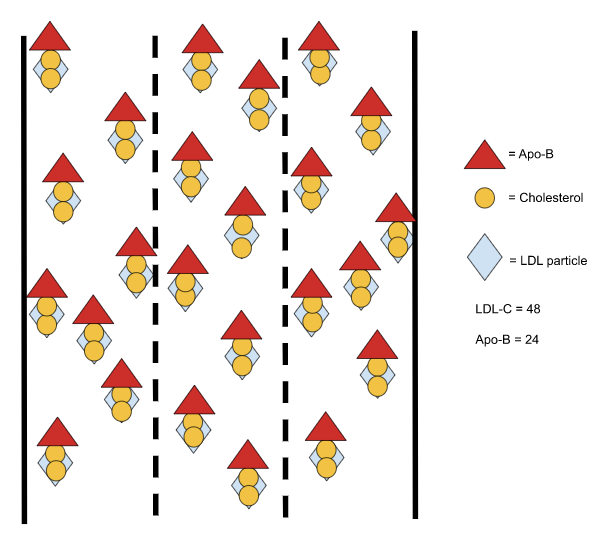
Dietary Cholesterol
As we discussed in the previous post, since much of the cholesterol we consume is esterified – meaning that it carries a bulky side-chain on it – and the Niemann-Pick C1-like proteins on our enterocytes (the cells that line our small intestine) filter out esterified cholesterol, we do not absorb most of the cholesterol that we eat. Although some epidemiology has associated dietary cholesterol with increased serum cholesterol for years, a recent secondary analysis of a 12-month long randomized controlled trial (RCT) strongly supports the idea that dietary cholesterol has a trivial, if any, impact on lipid metrics. Furthermore, The American Heart Association published a review in 2019 where they concluded that, “evidence from observational studies conducted in several countries generally does not indicate a significant association with [dietary cholesterol and] cardiovascular disease risk.”
Sauna
In 2015, Laukkanen et al. published a study showing that frequency of sauna use – divided by 1, 2-3, and 3-7 use(s) per week – progressively reduced CVD death and all-cause mortality in a sample of 2,315 Finnish men over the span of ~20 years. Specifically, 2-3 and 4-7 sauna sessions per week for a mean duration of ~14 minutes and at a mean temperature of ~78°C (~172°F) as compared to 1 use per week respectively demonstrated relative reductions of CVD mortality by 23% and 48% and all-cause mortality by 24% and 40% after adjusting for, “age, BMI, systolic blood pressure, serum low-density lipoprotein cholesterol level, smoking, alcohol consumption, previous myocardial infarction, type 2 diabetes mellitus, cardiorespiratory fitness, resting heart rate, physical activity, and socioeconomic status.”
The researchers themselves declared that the data, coming solely from middle-to-late aged Finnish men, is not necessarily generalizable to all people; furthermore, they only assessed sauna use at baseline – not throughout the entirety of the study – and sauna use could be indicative of other, “healthy,” lifestyle habits, so the conclusion is not concrete. With that said, I find the large sample size (n=2315), long follow-up duration(mean of 20.7 years), detailed adjustment for confounding variables, and both large and progressive relative risk reductions hard to ignore.
In addition to this study, experts in the field of longevity, such as Dr. Peter Attia (I) and Dr. Rhonda Patrick, have recently touted the growing pile of data supporting the health benefits of sauna. For the deepest dive I’ve seen on sauna use, check out Dr. Patrick’s summary of the literature and mechanisms associated with sauna use here.
Sleep
Multiple studies show relationships between sleep and CVD after heavily adjusting for other health, lifestyle, and demographic variables, demonstrating ~30-60% increases in relative risk of CVD for those sleeping less than 6 hours per night compared to those sleeping ~7-8 hours per night (I, II, III). Furthermore, in 2008, King et al. published data from their 5-year study that shows one additional hour of sleep per night confers a 33% decreased risk for coronary artery calcification – a sign of atherosclerosis development. Of course, all of this data is observational and cannot produce causal conclusions by nature; additionally, since sleep duration is likely a proxy for an overall healthy lifestyle, it is difficult to determine with certainty how much sleep duration alone affected CVD risk in these statistics. With that in mind, there is a large body of data showing that acute and chronic differences in sleep are important factors for cardiovascular health, glucose regulation, neurological health, and circadian health, many of which are discussed in this review, and all of which are addressed in Dr. Matthew Walker’s Why We Sleep.
Saturated Fat
Now, for the polarizing – or, as Dr. Peter Attia would call it, phosphorylating – question that everybody loves: how does saturated fat impact lipid metrics and CVD risk?
Before we dive into any of the numbers below, it is important to recognize the inherent fallibility of nutritional science, particularly that which aims to isolate the effects of single nutrients. There are many issues regarding design and execution of nutritional studies; however, I will use the following tangent to highlight 4 that I find to be particularly troublesome: dietary validation, trial duration, confounding, and subject blinding.
Firstly, the vast majority of nutritional studies rely upon food frequency questionnaires (FFQs) to acquire their data. I’ll demonstrate the problem with this tactic by asking you a question: what, how much, and when did you eat 4 days ago? How about every day for the past month, 6 months, or year? Unless you are a meticulous calorie-counting and macro-recording bodybuilder, then you are probably going to miss the mark of accurately reporting your consumption when filling out a FFQ. Even when studies instruct subjects to weigh and record their caloric intake, they often utilize a string of 4-7 days to represent the entirety of those subjects’ diets for 0.5-2 years – if the Hawthorne Effect bell isn’t ringing in your ear, it should be. For these reasons, one way or another, this inability to accurately record food intake curtails the strength of a lot of nutritional research.
Next, even when studies utilize clever protocols to track their subjects’ diets, like keeping them in a metabolic ward, providing all of their meals, or measuring blood ketone levels (a clever method in the case of a high-fat vs. low-fat trial) these steps typically limit the length of the trials, since it is difficult for the subjects to follow the instructed protocols for a long duration of time (i.e multiple months or years) – the issue of duration deepens when you consider the degree to which subjects remain in adherence with the dietary intervention over time. When looking at a drawn out process, like CVD, days to weeks is likely not enough time to capture at least some, if not many, of the desired signals.
Now, let’s suppose we conduct a well-designed trial with a large sample size, a long duration, and an accurate mechanism of determining the subjects’ food intakes. We are still not accounting for two important confounders: additional lifestyle factors and the replacement nutrients. The former is typically addressed through multivariate analysis – though these could never address all possible confounders – but the latter is of particular concern when looking at something like saturated fat for the following reason: if you see significant differences after altering saturated fat consumption, are those differences due to the removal of the saturated fat or the addition of the replacement nutrient? (i.e. if you aren’t lowering caloric intake, you have to replace the saturated fat with something, and you don’t know how adding that something is impacting the results) Add the issue of assessing the impact of changes in overall caloric intake, and things can get even messier.
Lastly, since subjects in nutritional RCTs know what they are eating every day, they cannot be blinded to which arm of the trial they are in. For example, each suject knows if they are in the high carb or low carb group, and this lack of blinding leaves the study vulnerable to the placebo effect.
Ok, now that I’ve finished my rant, and with all of that in mind, here are my findings regarding saturated fat consumption, Apo-B particle count, and CVD risk.
When it comes to saturated fat, it is easy to come across directly conflicting opinions, in that one party of low-fat and plant-based proponents may tell you that saturated fat is a one way ticket to the morgue; whereas, the other side, consisting of low-carb keto and carnivore fans, will tell you that saturated fat is irrelevant or even beneficial for CVD risk. If you traverse the vast literature around saturated fat yourself, it will become apparent that this issue is not as clean cut (pun-intended) as the vegetable chopper or steak slicer zealots suggest, in that the data show mixed results. In some cases, diets reduced in saturated fat (<10% of total calories) do reduce Apo-B count and/or CVD events/deaths (I, II, III, IV, V, VI); whereas, in other cases, the opposite appears to be true, in that diets higher in SFA (13% of total calories) outperform those low in SFA (4.9% of total calories) with regards to these metrics (I, II). In addition, there are many studies that show no significant differences in Apo-B count and/or CVD outcomes between those consuming high (13-20% of total calories) and low (<10% of total calories) amounts of SFA (I, II, III, IV, V, VI, VII, VIII, IX).
What explains this heterogeneity? One possibility is that these studies are failing to produce consistent, accurate, and clinically relevant data due to the previously mentioned design issues. Another explanation resides in the vast variability in human response to saturated fat consumption. Multiple studies (I, II, III, IV, V) have demonstrated this variability, together showing variations in LDL-C of +45% to -30% in response to increased saturated fat consumption, and some verifying that variance occurs in Apo-B response as well. This review from 2021 highlights some of this literature and notes some of the possible culprits, such as genetic variations in the APOE gene – responsible for encoding apoprotein E, an apolipoprotein, like Apo-B, that is found on VLDL and HDL and that, interestingly, is also suspected of strongly impacting Alzheimer’s Disease risk.
On top of this human variability, it is important to note that different saturated fats exhibit different effects on lipid metrics (I, II, III, IV, V), in that the, “shorter chain saturated fatty acids (6:0–10:0) and [stearic acid] have little effect on plasma cholesterol concentrations, whereas those with intermediate chain lengths (12:0–16:0) increase concentrations.” (I)
So, what conclusion can we draw from the jumbled data around saturated fat? I recently listened to Brad Schoenfeld Ph.D. – an expert in the fields of bodybuilding and muscular hypertrophy – describe the importance of incorporating scientific literature at the personal, N=1 level. “Research never will tell you what to do,” he said, “research gives you general guidelines, it basically gets you into the ballpark…Then you have to take personal expertise into account and take the needs and abilities of the individual [into account].” With something as slippery as saturated fat (again, pun intended), risk may be best determined from person to person using validated risk markers. With regards to the Apo-B Particle Model of Atherosclerosis, if saturated fat consumption increases your Apo-B count, then it is increasing your CVD risk; likewise, if saturated fat consumption decreases or does not impact your Apo-B count, then it is decreasing or not impacting your CVD risk respectively.